What is product usage analytics: Definition and guide for product managers
Last updated: March 2025
Product usage analytics describes the process of tracking and analyzing how users engage with your offering. It involves collecting data, studying user actions and product metrics, and uncovering insights that will inform your prioritization decisions. By analyzing product usage, you can better understand your end user behaviors. You can also see how the feature or functionality changes you make are impacting experiences and identify any friction points.
As a product manager, when you refer to product analytics you are likely thinking about quantitative metrics. For example, you might be assessing usage for a set of features or looking at data to see where new users drop off in your trial signup process. Product analytics can help eliminate guesswork by providing data-driven reference points to guide product decision-making.
Build products like you always wanted — start a free trial of Aha! software.
Keep in mind you will also need to gather qualitative feedback — from customer calls, support tickets, and an ideas portal. Together qualitative and quantitative data paint a full picture of the Complete Product Experience (CPE) so you can improve each touchpoint that customers have with your company and product.
Let's go deeper to learn more about the power of product analytics in informing strategic product decisions. Jump ahead using the links below:
What is the difference between product analytics and product metrics?
How to use product analytics to make better product decisions
Why is product analytics important?
The more customer input you can get, the better. Product analytics is one of the best (and most efficient) ways to collect customer data across a broad set of users. When you know exactly how users engage with your product, you can prioritize features and enhancements that will serve them best.
Product analytics can also inform how you define your product goals and initiatives. Analyzing user engagement can help you identify benchmarks, compare data across time, and determine product gaps that you need to address. Tying goals to measurable outcomes ensures that you can track the product team's progress towards meeting them.
Read more: How to make data-driven product decisions
Which teams use product analytics?
Product analytics is primarily used by product teams but it is useful for other teams, too. Cross-functional teammates collaborate with you to build, market, sell, and support your product. So it makes sense that they have a stake in product usage and want to know more about the value the product provides.
Here are some of the ways that teams across an organization use product analytics:
Customer support | To monitor engagement and inform customer conversations Product analytics clarify how to help customers use the product more efficiently and explore new functionality. |
Engineering | To identify areas of friction Engineers can hone in on which fixes to prioritize based on the level of user engagement. |
Executive team | To determine if product performance is on track to reach business goals Product metrics, like customer retention and revenue, are used as indicators of overall business success. |
Product management | To make decisions about the product roadmap — from product strategy to feature prioritization Quantitative data enriches your understanding of who your customers are and how they interact with your product. |
Product marketing | To better understand customer personas and how to reach them Product marketing teams can build engagement strategies by segmenting customers into groups based on common characteristics (like demographics or in-app behavior). |
UX and design | To identify areas of the user experience that can be improved UX managers pinpoint whether desired outcomes are being achieved by observing user actions like clicks and page views. |
What is the difference between product analytics and product metrics?
Analytics vs. metrics — the same thing? It sure sounds like they could be. And no one is likely to correct you if you mistake the terms. But if we are being precise here (and we like to be), here is how you can parse meaning:
Analytics is the information you can glean from the data
Metrics are the specific data points that inform your analysis
The metrics you choose to include within product analysis will vary based on your strategic planning process, industry, company size, and product type. In general, product metrics can be grouped into the following categories:
Business metrics: Data about company performance
Product usage metrics: Usage data that illuminates how users interact with your product or offering
Customer satisfaction: Metrics that help you understand whether customers are happy with their overall experience
Roadmap progress: Data about how the product team is progressing against the release timeline
Depending on the metrics you choose, it can be helpful to build a consolidated view of the data. The example below shows a product performance dashboard in Aha! Roadmaps — focused on progress towards strategic goals and delivering against the roadmap.
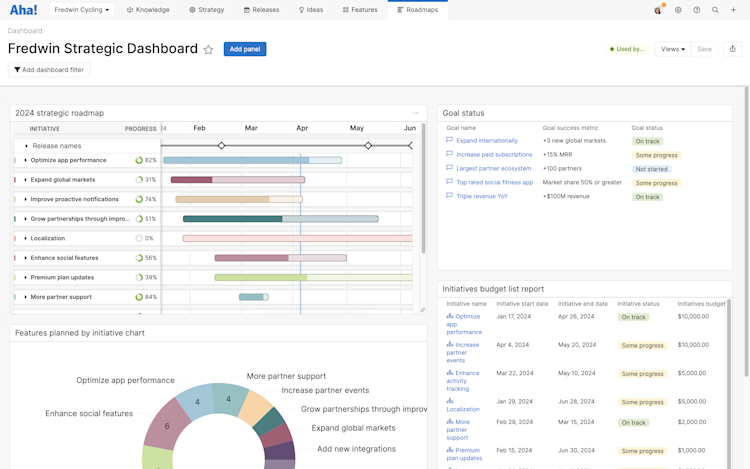
With Aha! software you can report on nearly every data element in your account then tailor your dashboard to include the exact information you need.
Related:
7 ways the Aha! team uses reports to show product development progress
McKinsey's take on what it means to be data-driven
Is product analytics the same as marketing analytics?
While product managers and marketing managers may both use analytics, what you track — and what you are looking to learn from the data — are different. Marketing analytics tends to focus on engagement with marketing campaigns and activities. This is often measured in views, conversion rates, trials, sales, add-ons, brand awareness, and share-of-voice.
That said, there will be some overlap. Both product and marketing teams care about revenue and growth rates as well as delivering value to customers. You should be united in shared goals and understand how each team's work contributes to the product and company vision.
How do product analytics tools work?
Product analytics tools capture data by tracking user actions within your product or website. Examples of user actions include clicks, page views, and signups. Product analytics tools help you understand how customers engage with your product by extracting insights from this data. Here are some common examples of product analytics tool capabilities:
Attribution analysis: Analyzing customer touchpoints (e.g., demos, sales conversations, website visits) that lead to purchase.
Churn analysis: Examining your customer loss rate to better understand what causes customers to leave.
Cohort analysis: Measuring behavioral patterns over time by separating users into related groups or cohorts.
Conversion analysis: Determining if customers are completing the desired conversion actions (e.g., signing up for a trial) or discovering where they drop off.
Funnel analysis: Mapping the customer journey through different stages that lead to a goal. This helps you understand points of friction or churn.
Retention analysis: Understanding the factors that entice your customers to stay (the inverse of churn analysis).
Segmentation: Dividing users into groups based on demographics, behavior, persona, and more to uncover deeper insights.
Related: Popular product analytics tools
When to invest in a product analytics tool
Because it is your primary tool for collecting, understanding, and visualizing your product data, it is a good idea to invest in product analytics whenever you have a viable product. The sooner you invest in product analytics, the more it will benefit you as your company grows — from informing your very first product launch to helping you retain important customers.
No matter which product analytics tool you choose, the overall objective should be the same — improve your product and customer experience. This means thinking broadly about what you are building and zeroing in on the data that brings the greatest insights. This is the mindset that leads to creating a product that customers love.
There are many product analytics tools on the market. Consider the product metrics you want to track, the types of analysis you intend to perform, necessary integrations, and budget to help you choose the best tool for your product and team.
How to use product analytics to make better product decisions
Data is plentiful. But deeply understanding what the data is telling you and taking action is more challenging. Numbers alone will not tell the full story. For example, if your paid account conversion rates are decreasing, you cannot immediately conclude that no one wants to buy your product. There could be something else in the signup experience that is causing users to abandon it.
So, how can you figure out what the data is telling you and then act upon it? To begin, you need to clearly define the questions you are trying to answer and tie them to the product goals you aim to achieve. Some of the most fundamental questions include:
How do customers find us?
Who are our most valuable customers?
What does product usage look like for our most valuable customers?
What causes customers to stop using our products?
For most organizations, it will take time to sufficiently answers these questions. It may require an investment in new data models or broadening the skill set of the team.
The table below includes additional questions to help you explore the meaning behind the metrics. Of course, you will want to supplement your analysis with questions of your own.
Attracting and acquiring customers |
|
Converting to paid customers |
|
Product usage and customer retention |
|
Team efficiency |
|
Remember that answering these types of questions requires quantitative data alongside qualitative discovery. This helps you get closer to the "why" behind the metrics and reveal truths that data alone may not uncover.
Try speaking directly to customers at different parts of the customer journey and collect qualitative data via interviews, ideas portals, empathy sessions, and surveys. With both product analytics and customer feedback in hand, you will be well-equipped to make meaningful improvements to your product.
Related: